New York City’s anti-bias law for hiring algorithms marks a significant step towards promoting fairness and preventing discriminatory biases in using AI-driven candidate screening tools. With the implementation of Local Law 144, NYC has taken a pioneering stance in addressing algorithmic bias and ensuring transparency in the hiring process. In this comprehensive article, we delve into the key aspects of the law, its implications for employers and job seekers, and the broader impact it may have on the future of AI-based hiring practices.
Addressing Bias in Hiring Algorithms
Implementing Local Law 144 highlights NYC’s commitment to addressing the potential biases embedded in AI-based hiring algorithms. Under this groundbreaking law, employers are prohibited from using automated employment decision tools for candidate screening unless the technology has undergone an independent bias audit within the past year. The law aims to promote fair and equitable hiring practices by mandating bias audits and disclosures, safeguarding candidates from potential discriminatory biases.
Key Provisions of Local Law 144
Local Law 144 encompasses several key provisions to ensure transparency and accountability in AI-driven hiring algorithms. Let’s explore these provisions in detail:
- Independent Bias Audits
Employers utilizing automated employment decision tools must undergo independent bias audits, which evaluate the algorithms for potential biases and discriminatory outcomes. These audits are vital in identifying and rectifying any biases in the A.I. systems used for candidate screening.
- Public Disclosure of Audit Results
To foster transparency, companies must publicly disclose the results of the bias audits they undergo. By making the audit findings accessible to the public, employers are held accountable for addressing biases and can demonstrate their commitment to fair hiring practices.
- Candidate Disclosures
Under Local Law 144, companies must provide clear disclosures to employees or job candidates regarding using automated hiring software. These disclosures should include a list of algorithms used and information about the “average score” candidates from different demographics will likely receive. By providing this information, candidates gain insight into the evaluation process and can better understand potential biases.
- Impact Ratios and Non-Compliance Penalties
The law defines “impact ratios” as a measure of algorithmic bias. Companies must be mindful of these impact ratios to ensure fairness in the hiring process. Non-compliance with the law’s provisions can result in penalties. This emphasis on accountability motivates employers to adhere to the guidelines and actively address biases in their hiring algorithms.
Implications and Broader Impact
The impact of NYC’s anti-bias law on hiring algorithms extends beyond the city’s borders. Implementing Local Law 144 sets a precedent for other regions, such as Washington, D.C., California, and New Jersey, where similar regulations are being considered to tackle bias in AI-driven hiring practices. Introducing such laws reflects the growing recognition of the need to address bias and discrimination in automated decision-making systems.
Moreover, the industry is actively exploring self-regulation through initiatives like the Data and Trust Alliance. These efforts aim to establish industry standards and guidelines that promote fairness and transparency in AI-driven hiring practices.
Ensuring Compliance and Mitigating Bias
Companies should adopt proactive measures to ensure compliance with Local Law 144 and mitigate bias in AI-based hiring practices. Here are some recommended steps:
- Robustness and Trustworthiness of Algorithms
Employers should prioritize the robustness and trustworthiness of their automated decision-making tools. This involves thorough testing, validation, and continuous monitoring of algorithms to identify and mitigate potential biases. Implementing rigorous quality control processes ensures the accuracy and fairness of algorithmic decision-making.
- Fairness Workflow
Following a fairness workflow can help organizations identify and address bias in their hiring algorithms. This workflow typically involves the following steps:
Bias Identification: Conduct an in-depth algorithm analysis to identify potential biases and discriminatory outcomes.
Root Cause Assessment: Understand the factors contributing to bias and assess the root causes, such as skewed training data or limited feature selection.
Bias Mitigation: Implement measures to mitigate bias, such as retraining models with diverse and representative datasets, adjusting algorithm parameters, or applying fairness-aware techniques.
Bias Reporting: Develop mechanisms to regularly evaluate and report on the fairness and performance of the algorithm. This ensures ongoing monitoring and accountability.
- Independent Auditors and Expertise
Engaging independent auditors with expertise in algorithmic fairness can provide valuable insights into evaluating and mitigating biases. These auditors can help organizations navigate the complexities of bias audits and offer guidance on best practices for fair and equitable hiring practices.
- Alternative Selection Processes
Businesses should be prepared to offer alternative selection procedures if candidates request it. Providing options that accommodate individual preferences can help ensure inclusivity and address concerns related to bias or discomfort with automated decision-making systems.
By proactively implementing these measures, organizations can comply with the law and contribute to creating a fair and inclusive hiring landscape.
Conclusion
New York City’s anti-bias law for hiring algorithms represents a significant step towards addressing algorithmic biases and promoting fairness in the hiring process. With provisions such as independent bias audits, public disclosures, and candidate transparency, the law emphasizes accountability and strives to eliminate discrimination in AI-based candidate screening. As other regions consider similar legislation, organizations must prioritize fairness, transparency, and compliance in their automated decision-making systems. By embracing these principles, companies can contribute to a more equitable and inclusive future of AI-driven hiring practices.
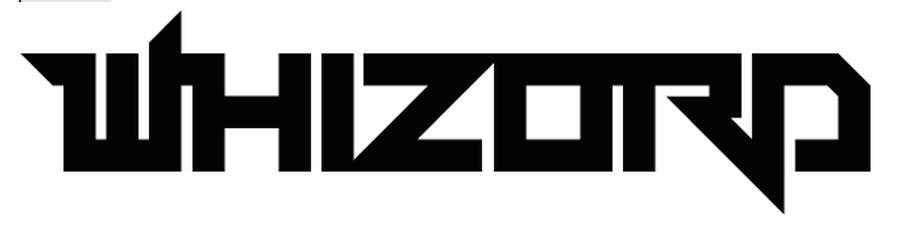