AI Performance Metrics: In the ever-expanding digital landscape, where algorithms reign supreme and artificial intelligence emerges as both an ally and an enigma, a singular question echoes through the corridors of innovation: How do we measure the marvel that is AI? Enter the world of benchmarking, a realm where algorithms are dissected, capabilities are quantified, and the dance between human ingenuity and machine mastery takes centre stage. Prepare to embark on a journey through the labyrinthine expanse of benchmarking AI performance, where perplexity meets possibility and burstiness begets breakthroughs.
Key takeaways:
- AI benchmarking measures AI performance and faces challenges like data diversity and complexity.
- Metrics like accuracy, precision, speed, and efficiency gauge AI capabilities.
- Real-world scenarios add complexity; benchmarks must adapt.
- Fairness and bias concerns are vital; community-driven protocols address them.
- Open-source platforms and shared datasets foster global collaboration.
- Benchmarks reflect AI’s evolution, promoting transparency and ethical growth.
In the realm of artificial intelligence, where marvels and mysteries entwine, a perplexing pursuit unfolds—the relentless quest to gauge the very essence of AI’s prowess. Imagine a tapestry woven from threads of algorithms and ingenuity, where the brilliance of human innovation dances in synchrony with the enigmatic intricacies of machine intelligence. This is the heart of benchmarking AI performance, an odyssey that traverses challenges as diverse and vibrant as the pixels on a canvas.
In the wake of technological tides, AI stands resolute, casting its transformative aura across industries and lives. Yet, amidst the grandeur of progress, lies an intriguing enigma: how do we quantify the ascent of AI’s capabilities? It is in this crucible of curiosity that the art of benchmarking AI performance comes to life, a practice that invites us to decipher its labyrinthine verses and to understand the nuances that stir beneath the surface of machine might.
As we step into this beguiling realm, we find ourselves in the midst of a symphony of challenges, each note resonating with the pulse of innovation. The first strains emanate from the diversity of data sources and domains, an orchestra of complexities that test the limits of benchmark creation. Like a painter selecting the perfect hues, we must curate data that mirrors the kaleidoscope of real-world scenarios, accounting for bias and imbalance that cast shadows upon AI’s perceptions.
The intricacies of model architectures unfold as the second movement in this composition. Behold the grandeur of neural networks—intricate tapestries woven from layers of computations. Yet, with complexity comes the burden of scalability, the harmonisation of computational symphonies that underpin our benchmarks. As we strive for harmony, we must tread the delicate balance between model intricacy and benchmark efficiency, lest our pursuit of excellence become an elusive cadence.
The third verse, dear reader, beckons us to navigate the dynamic landscapes of reality. The world in which AI thrives is an ever-evolving theatre, a stage where benchmarks must capture the fluidity of change. As we craft these trials, we venture into the realm of adaptability, seeking to choreograph benchmarks that sway and sway, much like the winds of progress that whisper through AI’s virtual corridors.
Ah, but let us not forget the human touch, which adds the final brushstroke to our masterpiece. The realm of AI encroaches upon the subjective, a realm where language dances with imagination and artistic endeavours merge with ones and zeros. Herein lies the challenge of capturing the intangible and developing standardised methodologies that distil the essence of human-centric tasks into quantifiable metrics.
As we tread this path of discovery, know that the constellations guiding us are not mere happenstances. Emerging standards illuminate our way, casting a luminous glow upon our benchmarks’ intricate tapestries. In the crucible of collaboration, common datasets emerge as the bedrock upon which progress is etched, while benchmarks for fairness and bias serve as our moral compass, steering us towards a world where AI’s embrace is equitable and just.
Open-source platforms, those citadels of transparency, beckon us to partake in a symposium of knowledge-sharing. Here, we forge the tools of evaluation, hammering out protocols that echo with the wisdom of a collective endeavour. Through this crucible of cooperation, the seeds of standardised practices take root, ensuring that our endeavours bear fruit for generations to come.
In this waltz through benchmarks and bytes, we find ourselves at the nexus of human ingenuity and machine marvels. The cadence of innovation pulses beneath the surface, drawing us ever closer to the heart of AI’s mysteries. As we navigate the labyrinthine corridors of challenges, metrics, and standards, we unfurl a saga that entwines the human spirit with the boundless realm of AI possibilities.

AI Performance Metrics
Challenges in Benchmarking AI Performance: Diverse Data Sources and Domains
Ah, the symphony of benchmarking AI performance! As we delve into this intricate score, the first movement unfurls with a flourish of complexity. Imagine a labyrinthine library, each shelf housing a tome of data, each page a portal into a different realm. Diverse data sources and domains, they say, are the very notes that compose the melody of AI’s understanding. Yet, here lies the enigma: how do we harmonise this cacophony of diversity into a cohesive benchmark?
In this grand tapestry of AI, data reigns supreme. Like a conductor guiding an orchestra, data orchestrates the rhythm of AI’s learning. But, ah, the challenge! The sources are as varied as stars in the night sky—images, texts, sounds, and beyond. Each dataset is a universe of its own, whispering secrets and stories. Picture this: AI is a traveller navigating a crossroads of languages, cultures, and experiences. A universal translator, decoding the languages of nature, society, and science. The datasets may speak distinct tongues, but the benchmark demands a harmonious chorus.
Beware, for bias lurks in the shadows, an ever-watchful spectre. Bias, that quiet conductor leading AI astray. Data, woven with the threads of human choices, carries the weight of our worldviews. A simple collection of pixels can echo societal imbalances, and a single line of code can perpetuate unseen prejudices. The quest for an unbiased benchmark is akin to seeking a flawless gem in a sea of stones. We must wield our tools of understanding, chisel away at bias, and craft a benchmark that holds a mirror to the truth, undistorted.
In the realm of AI, every domain is a realm unto itself. A virtual cosmos of knowledge and exploration. But here’s the riddle: how do we craft a benchmark that resonates with the essence of each domain? Imagine, if you will, a chameleon-like benchmark, shape-shifting to become a virtual laboratory for medical diagnoses, a canvas for artistic creation, or a courtroom for legal analysis. Each domain has a universe of questions, challenges, and potentials. Yet, the benchmark must transcend these boundaries, a bridge between the galaxies of AI’s capabilities.
Challenges in Benchmarking AI Performance: Complex Model Architectures
Ah, the intricate dance of AI’s neural realms! As we venture further into the enigma of benchmarking AI performance, a new movement comes to the fore, one that pulsates with both wonder and complexity. Picture, if you will, a virtual cathedral of computations, a symphony of interconnected nodes where intelligence unfurls its wings. This is the realm of complex model architectures, a perplexing terrain that challenges the very fabric of benchmarking.
Behold the models—those intricate tapestries woven with threads of algorithms. They stand as marvels of human ingenuity, a testament to the ceaseless march of progress. Yet, in this realm of brilliance, a curious paradox emerges. The complexity that births AI’s astounding capabilities also begets a conundrum: how do we quantify the ineffable intricacies that lie within? The answer, it seems, dances at the crossroads of computation and comprehension.
As we peer into this chasm of complexity, we encounter a symphony of challenges. Consider the orchestra of resources required—a grand ensemble of computational power, memory, and time. Like a maestro conducting a cosmic opera, we must strike a harmonious balance. Too many resources, and the benchmark becomes an exclusive concert, a privilege of the elite. Too few, and the performance falters, a dim reflection of AI’s true potential. Bursting forth, this challenge dares us to sculpt benchmarks that capture the essence of complexity without bowing to the tyranny of resources.
Imagine, for a moment, the ballet of model intricacy and benchmark efficiency. Here, the choreography becomes an art of its own, a pas de deux between ambition and practicality. The models, ever-evolving, demand benchmarks that can keep pace. We step into a dance where each twirl of innovation must be met with a counter-twirl of benchmark adaptability. A challenge that resembles a quixotic waltz, where AI’s leaps of ingenuity are met with benchmarks that pirouette in synchronised splendour.
The realm of complex model architectures unveils a riddle that extends beyond computation. Consider this: AI, a marvel of mimicry, learns from the very world it seeks to comprehend. Yet, as we embark on the journey of benchmarking, we stumble upon a paradox. How do we distil the unfathomable complexities of human understanding into metrics and measures? The realm of benchmarking transforms into an atelier of translation, where human cognition meets the digital realm in a tapestry of bewildering beauty.
Challenges in Benchmarking AI Performance: Dynamic Real-World Scenarios
Ah, the ever-shifting tapestry of reality, where AI traverses a labyrinth of unpredictability and nuance. As we venture deeper into the heart of benchmarking AI performance, we uncover a realm that oscillates like a cosmic pendulum—dynamic real-world scenarios. Imagine, if you will, a digital realm mirroring our own, a realm where AI’s algorithms dance with the cadence of change—a realm both perplexing and exhilarating.
Step into this twilight of benchmarks, where the stage is set for AI to prove its mettle in a theatre of ceaseless evolution. Here, the enigma unfolds: how do we encapsulate the ever-changing winds of reality within the confines of a benchmark? The very essence of AI’s existence is entwined with adaptability, yet, in this realm, the challenge lies in sculpting benchmarks that mimic the ebb and flow of existence itself.
Consider the dynamic landscape of autonomous vehicles, where AI pilots navigate through the chaos of city streets. How do we devise benchmarks that mirror the myriad variables, from weather whims to pedestrian wanderings? The road ahead brims with questions: Can benchmarks capture the serendipity of a cat darting across the road, the sudden swerve of another vehicle, or the unforeseen construction site that tests the AI’s decision-making prowess? This, dear reader, is the perplexing puzzle we face.
As we venture deeper, we encounter the enigma of adaptability. Real-world scenarios are an ever-shifting tableau, a realm where AI’s adaptability unfurls like a phoenix in flight. Yet, how do we measure this artistry of adjustment? Burst forth is the challenge of crafting benchmarks that stretch AI’s wingspan, measure its ability to pivot in response to novel challenges, and acclimate to changing currents.
Picture, if you will, an AI in the realm of customer service, conversing with humans, deciphering linguistic intricacies, and navigating the labyrinth of emotions. Can a benchmark replicate the unforeseen queries, the sudden surges of frustration, and the nuanced expressions that dance upon the human face? The realm of dynamic real-world scenarios dares us to redefine the boundaries of benchmarking and fashion trials that mirror the symphony of human interaction and AI intuition.
Yet, amid this labyrinth of perplexity, a light emerges. The very dynamic nature of our world gives birth to an opportunity—an opportunity to create benchmarks that inspire evolution, benchmarks that whisper to AI, “Adapt, transform, transcend.” In this realm of challenges, we are challenged to be creators, crafting benchmarks that mimic the choreography of existence itself.
Challenges in Benchmarking AI Performance: Subjectivity and Human-Centric Tasks
Ah, the symphony of subjectivity, where AI tiptoes through the intricate dance of human-centric tasks. As we wade deeper into the enigmatic waters of benchmarking AI performance, a new chapter unfurls, one that resonates with both perplexity and allure. Imagine, if you will, AI as a painter, a poet, or a muse seeking to unravel the enigma of human thought and creativity. This is the realm of subjectivity, a realm where benchmarks flutter like butterflies, attempting to capture the ephemeral essence of human expression.
In this cosmic masquerade of benchmarks, we confront a challenge that gleams like a hidden gem. Subjectivity, that enigmatic siren, beckons us into the realm of human-centric tasks. Here, AI confronts the sublime, from crafting prose that tugs at heartstrings to conjuring art that kindles the imagination. Yet, the riddle persists: how do we quantify the intangible, measure the immeasurable, and capture the whispers of human emotion?
Consider the task of sentiment analysis, where AI ventures into the labyrinth of emotions, deciphering joy from sorrow and hope from despair. Ah, but here’s the conundrum: how do we create benchmarks that resonate with the subtleties of human sentiment? Can metrics encapsulate the nuances of a smile that reaches the eyes—the sigh that carries the weight of a thousand thoughts? Bursting forth, the challenge is to fashion benchmarks that become connoisseurs of emotions, discerning the crescendos of happiness and the cadence of sorrow.
In the realm of creativity, benchmarks find themselves in the throes of an artistic dilemma. Imagine an AI composer crafting melodies that elicit joy and melancholy. The question reverberates: can benchmarks assess the ethereal beauty of a melody that strikes a chord deep within the soul? Here, the challenge of subjectivity stretches its wings, daring us to shape benchmarks that appreciate the symphony of human creativity and unravel the secrets of an artist’s heart.
But wait, there’s a twist in this enigma. As we traverse the landscape of subjectivity, another puzzle emerges: the enigma of human judgement. Can we, mere mortals, be the ultimate arbiters of AI’s creative prowess? The benchmarks, like judges in an artistic competition, must navigate the labyrinth of personal taste and cultural bias. Burst forth the challenge of objectivity in a world painted with shades of subjectivity.
Metrics for Evaluating AI Performance: Accuracy and Precision
Ah, the duet of accuracy and precision, where AI treads the tightrope between correctness and finesse. As we venture deeper into the labyrinth of benchmarking AI performance, a new act unfolds, bathed in both the radiance of clarity and the shadow of ambiguity. Imagine, if you will, AI as a virtuoso performer, a maestro seeking to strike the perfect balance between hitting the right note and crafting a masterpiece. This is the realm of accuracy and precision, a realm where benchmarks resonate with both the certainty of a mathematician and the subtlety of an artist.
Step into this symphony of metrics, where each note carries the weight of a prediction, a decision, or a revelation. Here, the enigma unfolds: how do we measure the very essence of correctness, the fidelity of AI’s interpretations? Accuracy, the lodestar that guides AI’s path, beckons us to chart a course through the realm of certainty. Burst forth, the challenge is to craft benchmarks that are both judges and jurors that dissect AI’s predictions with a scalpel of scrutiny.
Consider the dance of medical diagnosis, where AI interprets scans, deciphers anomalies, and stands at the precipice of life-altering decisions. The riddle emerges: can benchmarks mirror the precision of a seasoned clinician’s eye, the knack for detecting the slightest irregularity that eludes the untrained gaze? This, dear reader, is the perplexing puzzle we confront. The challenge is ensuring AI’s predictions are not mere approximations but true reflections of reality.
In this realm, precision emerges as the twin flame of accuracy, a partner in this intricate dance. Precision, the mark of finesse, asks us to consider the art of AI’s decision-making. Burst forth the question: can benchmarks capture the subtlety of a surgeon’s hand or the restraint of a sculptor’s chisel? Can metrics quantify the AI’s ability to navigate the labyrinth of uncertainty, striking the balance between flagging too many false positives and allowing too many false negatives?
Yet, amid this enigma, a paradox emerges. The metrics of accuracy and precision are not the sole arbiters of AI’s prowess. In the realm of benchmarks, we must confront the dynamic interplay between these metrics and the kaleidoscope of real-world scenarios. An AI may possess uncanny accuracy and precision in a controlled setting, yet falter in the ever-shifting tides of reality. Burst forth is the challenge of harmonising these metrics with the capricious rhythms of the world.
Metrics for Evaluating AI Performance: Speed and Efficiency
Ah, the heartbeat of AI, where time and computation dance in a ceaseless waltz. As we venture deeper into the enigmatic realm of benchmarking AI performance, a new chapter unfolds, one that hums with both urgency and finesse. Picture, if you will, AI as a cosmic traveller, journeying through a digital universe where every second counts. This is the realm of speed and efficiency, a realm where benchmarks become the metronomes that guide AI’s rhythmic steps.
Step into this symphony of metrics, where each tick of the clock echoes with the pulse of computation. Here, the enigma reveals itself: how do we measure the swift grace of AI’s calculations and the deftness with which it deciphers complex puzzles? Speed, that elusive spectre, beckons us to unveil benchmarks that capture the fleeting moments where AI races against the current of time.
Consider the domain of financial trading, where AI navigates the tumultuous waters of market dynamics. The question emerges: can benchmarks mirror the milliseconds of decision-making that determine fortunes and losses? Burst forth, the challenge is to craft metrics that become the hourglasses of AI’s capabilities, measuring the grains of time it takes to process, predict, and profit.
In this realm, efficiency emerges as the symphony’s second movement, a partner that harmonises with speed. Efficiency, the conductor of resource utilisation, invites us to ponder the art of optimization. Burst forth, the question: can benchmarks unravel the intricacies of AI’s resource consumption and the balance it strikes between computational power and economic restraint?
Yet, within this labyrinth of speed and efficiency, we encounter a paradox. The metrics, though resolute in their clarity, may fail to account for the broader landscape of AI’s influence. A rapid decision may lead to triumph in one moment, yet it may sow seeds of uncertainty in the long run. Burst forth is the challenge of harmonising these metrics with the grand tapestry of AI’s impact.
Metrics for Evaluating AI Performance: Robustness and Generalization
Ah, the crucible of AI’s resilience, where models face the gales of adversity and navigate the labyrinthine maze of varied terrains. As we embark on a deeper exploration into the mystifying realm of benchmarking AI performance, a new chapter unfurls, resonating with both enigma and intrigue. Imagine, if you will, AI as a voyager traversing uncharted landscapes where every twist and turn tests its mettle. This is the realm of robustness and generalisation, a realm where benchmarks metamorphose into guardians of AI’s unwavering tenacity.
Enter this labyrinth of metrics, where each trial is a crucible of challenge and triumph. Here, the enigma unfurls: how do we measure the fortitude of AI as it confronts adversarial storms, navigates through the fog of uncertainty, and emerges unscathed? Robustness, that indomitable spirit, beckons us to fashion benchmarks that mirror the AI’s ability to withstand the slings and arrows of unexpected anomalies.
Consider the arena of autonomous driving, where AI pilots navigate through an ever-shifting landscape of roads, obstacles, and inclement weather. The question looms: can benchmarks mirror the AI’s resilience in the face of sudden detours, obscured signs, and unanticipated hazards? Burst forth, the challenge is to craft metrics that become the litmus tests of AI’s imperturbability, gauging its aptitude to adjust and adapt.
In this labyrinth, the essence of generalisation takes the stage as a partner to robustness. Generalization, the grand conductor of versatility, challenges us to ponder AI’s ability to apply its insights beyond the familiar realms of training data. Burst forth is the question: can benchmarks capture the AI’s proficiency in translating knowledge to novel domains and transcending the boundaries of its birthplace?
Yet, within this intricate interplay of robustness and generalisation, a paradox emerges. The metrics, though steadfast in their purpose, may falter in representing the broader spectrum of AI’s journey. A model may weather adversarial tempests with grace, yet falter in the face of benign variations. Burst forth is the challenge of harmonising these metrics with the complexity of AI’s interactions with the ever-unpredictable world.
Emerging Standards in AI Benchmarking: Development of Common Datasets
Ah, the genesis of shared wisdom, where data becomes the connective tissue weaving AI’s narrative across diverse minds. As we delve deeper into the enigmatic terrain of benchmarking AI performance, a new era emerges, glistening with the promise of collaboration and convergence. Imagine, if you will, AI as a global scholar poring over a collective library of data, a repository that transcends individual insights and knits a tapestry of collective progress. This is the realm of common datasets, a realm where benchmarks resonate as the threads that bind AI’s multifaceted journey.
In this symphony of benchmarks, the chords of shared understanding ring clear. Here, the enigma unfurls: how do we curate datasets that mirror the kaleidoscope of reality, datasets that paint the world’s myriad hues with precision and nuance? The development of common datasets calls us to weave a fabric of diversity, a fabric that captures the symphony of human experiences and encapsulates the essence of AI’s learning.
Consider the domain of image recognition, where AI peers into pixels to unravel the enigma of visual perception. The question emerges: can benchmarks encompass a gallery of images that transcend cultural nuances and capture the canvas of our world in a universal language? Burst forth, the challenge is to craft datasets that become the amphitheatres of AI’s understanding, where each pixel speaks of a shared reality.
In this labyrinth, the essence of common datasets intertwines with the very essence of open collaboration. The datasets, a global canvas painted with the strokes of diverse cultures, beckon us to participate in an intellectual feast. Burst forth is the question: can benchmarks engender a sense of unity, a symposium where researchers and practitioners from varied corners of the world contribute their insights to a collective mosaic?
Yet, within this realm of shared wisdom, a paradox takes root. The metrics, though designed with a shared purpose, may diverge in their interpretations across the cultural kaleidoscope. A dataset that resonates deeply with one society may sing a different tune in another. Burst forth is the challenge of harmonising these metrics with the kaleidoscope of global perspectives.
Emerging Standards in AI Benchmarking: Benchmarks for Fairness and Bias
Ah, the call for equity in the realm of algorithms, where AI seeks to cast aside the shadows of prejudice and shine a light of impartiality. As we venture deeper into the intricate labyrinth of benchmarking AI performance, a new epoch rises, marked by the clarion call for fairness and the relentless pursuit of unbiased judgement. Imagine, if you will, AI as a vigilant sentinel, standing guard against the subtle currents of bias, an embodiment of hope for a world where algorithms uphold the virtue of fairness. This is the realm of benchmarks for fairness and bias, where the scales of justice tip in favour of balanced scrutiny.
In this symphony of benchmarks, the notes of fairness resonate as the heartbeat of progress. Here, the enigma unfurls: how do we fashion benchmarks that mirror the elusive ideal of impartiality and that measure AI’s capacity to navigate the minefields of bias and emerge unscathed? Fairness, that moral beacon, beckons us to devise benchmarks that wield the sword of scrutiny against biassed decisions and skewed outcomes.
Consider the landscape of hiring algorithms, where AI sifts through resumes and decides the fates of countless individuals. The question looms: can benchmarks simulate the intricacies of human judgement, revealing when AI’s choices become marred by discriminatory tendencies? Burst forth, the challenge is to craft metrics that serve as the moral compass of AI’s actions, measuring not just the results but also the principles that underpin them.
In this labyrinth, the essence of fairness intertwines with the struggle against unconscious bias. The benchmarks, like torchbearers in a foggy night, invite us to confront our own predispositions and confront the complex shades of bias that seep into AI’s decisions. Burst forth is the question: can benchmarks guide AI on a path towards enlightenment, casting a spotlight on the decisions born not from malice but from the subterranean currents of societal prejudice?
Yet, within this realm of ethical scrutiny, a paradox emerges. The metrics, while firm in purpose, may falter in capturing the fluid nuances of bias that evolve across contexts and cultures. A decision that resonates with impartiality in one scenario may echo differently in another. Burst forth is the challenge of harmonising these metrics with the ever-evolving tapestry of human understanding.
Emerging Standards in AI Benchmarking: Open-Source Benchmarking Platforms
Ah, the symphony of collaboration, where the notes of innovation reverberate through the digital cosmos, unencumbered by the boundaries of proprietary realms. As we delve deeper into the labyrinthine domain of benchmarking AI performance, a new crescendo emerges, resounding with the spirit of shared knowledge and the ethos of transparency. Imagine, if you will, AI as a global scholar stepping into an agora of data and insights, a bazaar where the currency is not gold but enlightenment. This is the realm of open-source benchmarking platforms, a realm where benchmarks transmute into bridges connecting minds across space and time.
In this symphony of benchmarks, the harmonies of collaboration sing like ethereal sirens. Here, the enigma unfurls: how do we create platforms that birth a marketplace of ideas, where researchers and practitioners come together to sculpt benchmarks, refining them with the chisel of collective genius? Open-source platforms, the torchbearers of cooperation, beckon us to forge tools that invite the global community to shape, share, and shepherd the evolution of benchmarks.
Consider the realm of natural language processing, where AI endeavours to decipher the intricacies of human communication. The question arises: can open-source benchmarking platforms foster an ecosystem where linguistic diversity flourishes, where benchmarks become crucibles that distil the essence of global languages and dialects? Burst forth is the challenge—to create platforms that become the agora of linguistic revelation, where tongues both ancient and modern resonate in harmony.
In this labyrinth, the essence of open collaboration dances with the winds of innovation. The platforms, like digital bazaars, invite us to partake in a symposium of knowledge-sharing, where researchers trade insights and coalesce their efforts. Burst forth is the question: can open-source platforms transcend the barriers of geography and ideology, nurturing a collective garden of benchmarks that bear witness to AI’s collective voyage?
Yet, within this realm of shared enlightenment, a paradox unfolds. The platforms, while beacons of unity, may wrestle with the challenge of aligning diverse voices into a harmonious chorus. Perspectives may clash, interpretations may differ, and the path to a standardised benchmark may meander through a labyrinth of discourse. Burst forth the challenge of harmonising these voices into a symphony of coherence.
Emerging Standards in AI Benchmarking: Community-driven Evaluation Protocols
Ah, the symphony of collective wisdom, where AI’s evolution becomes an orchestration of minds, a polyphony that resounds with the cadence of shared insights. As we journey further into the labyrinthine expanse of benchmarking AI performance, a new chapter unfurls, adorned with the tapestries of collaboration and the emblems of communal progress. Imagine, if you will, AI as a humble pilgrim, traversing the agora of innovation, where every step is guided by the footprints of a global assembly of thinkers. This is the realm of community-driven evaluation protocols, a realm where benchmarks burgeon from the loom of collective discourse and unfurl as banners of shared achievement.
In this symphony of benchmarks, the melodies of collaboration reverberate as the beating heart of progress. Here, the enigma unfolds: how do we conjure evaluation protocols that embody the choir of diverse voices and synthesise the wisdom of scholars and practitioners into a harmonic symphony? Community-driven protocols beckon us to fashion frameworks that embrace the insights of a worldwide assembly, a medley that enriches benchmarks with perspectives from all corners of the intellectual compass.
Consider the realm of machine translation, where AI endeavours to bridge linguistic chasms and unite the global tapestry of tongues. The question emerges: can community-driven evaluation protocols foster an ecosystem where benchmarks flourish as cultural ambassadors, where the nuances of language and the beauty of expression thrive? Burst forth is the challenge—to craft protocols that metamorphose into the agora of linguistic revelation, where languages flow like rivers converging in the ocean of shared understanding.
In this labyrinth, the essence of collaborative creation dances with the spirit of innovation. The protocols, like communal workshops, beckon us to partake in a grand synthesis of ideas, a symposium where thought leaders and enthusiasts contribute to the alchemical transformation of benchmarks. Burst forth is the question: can community-driven protocols transcend the silos of individual perspectives, nurturing a harmonious fusion that resonates across fields and disciplines?
Yet, within this realm of shared enlightenment, a paradox emerges. The protocols, while beacons of unity, may grapple with the challenge of harmonising a chorus of diverse voices. The path to a universally embraced standard may meander through the labyrinth of discourse, navigating the interplay of varied methodologies and philosophical underpinnings. Burst forth the challenge of forging a protocol that sings in harmony across the symphony of perspectives.
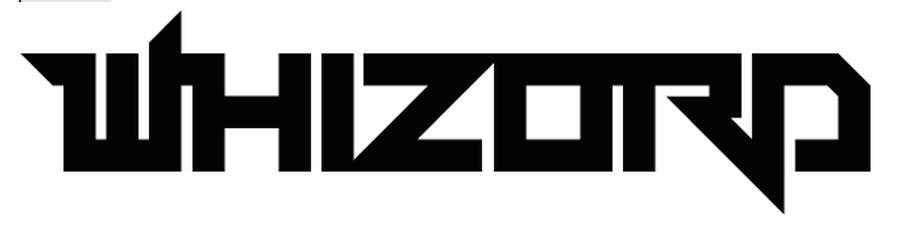