Explore the environmental impact of developing and deploying AI systems. Learn how AI affects your planet. Environmental impact, AI systems, developing and deploying.
KEY TAKEAWAYS
- AI development uses a lot of energy, so making AI more energy-efficient is crucial for the environment.
- Rapid hardware upgrades in AI contribute to e-waste, highlighting the need for sustainable hardware practices and recycling.
- To protect the environment, it’s vital to balance AI innovation with eco-friendly principles.
In your era of relentless technological advancement, the rise of AI systems has brought remarkable changes to your lives. From smart assistants to autonomous vehicles, AI has revolutionized the way you work and play. However, amid the excitement of these innovations, a critical concern looms large: the environmental impact of developing and deploying AI systems. This article delves deep into the hidden costs that often go unnoticed. you’ll explore the astonishing energy appetite of AI, the challenges posed by electronic waste in rapidly evolving hardware, and the intricate balance between technological progress and environmental preservation.
Uncover the lesser-known facets of AI’s footprint on your planet. Discover how the choices made in developing and deploying AI systems resonate far beyond the digital realm, affecting your environment in profound ways. The strategies and initiatives aimed at creating a more sustainable future in the world of AI will also be highlighted.
Understanding AI Systems
Artificial Intelligence (AI) is reshaping the world around us, from how you interact with technology to how businesses operate and even how you address environmental challenges. Before delving into AI’s environmental impact, it’s essential to understand the various types and applications of AI.
Machine Learning
Machine Learning (ML) is a subset of AI that focuses on enabling systems to learn and improve from experience without being explicitly programmed. ML algorithms analyze large datasets to identify patterns, make predictions, and continuously refine their models. This technology has applications ranging from image recognition to climate modeling.
Deep Learning
Deep Learning, a subset of ML, involves artificial neural networks inspired by the human brain’s structure. Deep Learning excels in tasks such as speech recognition, autonomous driving, and medical image analysis. However, its computational demands can have significant environmental implications, as training deep neural networks requires substantial energy resources.
Natural Language Processing
Natural Language Processing (NLP) enables AI systems to understand and generate human language. This technology is behind chatbots, language translation, and sentiment analysis. NLP applications can enhance communication and data analysis but also involve resource-intensive computations.

Photo: Kacper Pempel
AI Development Life Cycle
Understanding the AI development life cycle is crucial for comprehending how AI systems impact the environment at different stages of their creation and deployment.
Data Collection and Preprocessing
The AI journey begins with data collection and preprocessing. Massive datasets are gathered, cleaned, and prepared for analysis. Data collection processes can have environmental impacts, especially when they involve resource-intensive activities like data centers and data storage.
Model Training
Model training is where AI systems learn from data. It often demands substantial computational power, which translates into energy consumption. Training sophisticated models, such as those used in deep learning, can be particularly energy-intensive, contributing to the overall environmental footprint.
Deployment and Post-Deployment Phases
Once trained, AI models are deployed for various applications, which can include optimizing energy usage, predicting environmental changes, or even monitoring wildlife populations. The deployment phase may require hardware infrastructure, and its environmental impact depends on factors like efficiency and sustainability.
Understanding these facets of AI systems is essential to appreciate how their development and deployment impact the environment.
Environmental Impact Factors
As the development and deployment of AI systems continue to expand, it’s imperative to assess their environmental impact. This section explores the key factors contributing to AI’s environmental footprint.
Energy Consumption
Energy consumption is a paramount concern when evaluating the environmental impact of AI systems. The following subheadings dissect this aspect:
Training and Inference Energy
Training AI models, particularly deep learning models, requires immense computational power. This energy-intensive process involves running calculations on powerful GPUs or TPUs over extended periods. The environmental consequences stem from electricity consumption, often derived from fossil fuels.
Hardware Infrastructure
The infrastructure supporting AI development, including data centers and high-performance computing clusters, demands significant energy resources for cooling and maintenance. Energy-efficient data centers and hardware play a pivotal role in mitigating these impacts.
E-Waste and Hardware Lifecycle
Rapid Hardware Obsolescence
The rapid pace of AI advancement leads to hardware obsolescence at an unprecedented rate. AI researchers and organizations frequently upgrade their equipment to keep up with evolving AI models. This continual turnover contributes to electronic waste (e-waste) and poses environmental challenges.
Recycling Challenges
Recycling AI hardware presents unique challenges due to the complexity of components and the rapid evolution of technology. Proper disposal and recycling practices are essential to reduce the environmental impact of discarded AI hardware.

Photo: Kacper Pempel
Carbon Emissions
AI Data Centers
Data centers, where massive AI computations take place, are major contributors to carbon emissions. The electricity consumed by these data centers, often powered by fossil fuels, results in substantial greenhouse gas emissions.
Transportation for AI Hardware
The transportation of AI hardware components, such as GPUs and specialized chips, adds another layer of carbon emissions. The global supply chain involved in manufacturing and distributing these components contributes to the environmental footprint of AI.
Understanding these environmental impact factors is crucial for developing strategies to reduce AI’s negative effects on your planet.
Case Studies and Real-World Examples
To gain a deeper understanding of the environmental impact of developing and deploying AI systems, some real-world case studies and initiatives are examined.
Prominent AI Projects and Their Environmental Impact:
This section will spotlight a few notable AI projects and their environmental implications, shedding light on the tangible effects of AI on your planet.
Autonomous Vehicles and Energy Consumption
Autonomous vehicles, a frontier of AI, promise to revolutionize transportation. However, the energy-intensive computations required for self-driving systems raise concerns about increased fuel consumption and emissions. Analyzing their environmental trade-offs is crucial as these technologies mature.
Precision Agriculture and Sustainable Farming
AI-driven precision agriculture optimizes crop management, reducing resource wastage and improving yields. This approach, enabled by AI, minimizes the environmental impact of farming by efficiently using water, fertilizers, and pesticides.
Climate Modeling and Predictive Analytics
Climate modeling, powered by AI, aids in understanding climate change and making accurate predictions. These models help scientists and policymakers assess the environmental impact of various scenarios and develop strategies for mitigation.
Companies’ Initiatives in Green AI
Many tech companies are recognizing the need for sustainable AI development. This subsection explores how these companies are taking steps to address the environmental concerns associated with AI.
Energy-Efficient AI Hardware
Some companies are designing energy-efficient AI hardware, reducing the power consumption of AI systems. These innovations contribute to a more sustainable AI ecosystem.
Renewable Energy Usage in Data Centers
Tech giants are increasingly powering their data centers with renewable energy sources, such as solar and wind. Shifting to cleaner energy options significantly reduces the carbon footprint of AI computations.
Carbon Offsetting and Environmental Responsibility
Several companies are investing in carbon offsetting initiatives to neutralize the emissions generated by their AI operations. These efforts showcase a commitment to environmental responsibility.
By examining these case studies and initiatives, you can better appreciate both the challenges and opportunities that AI presents in the context of environmental impact. It’s evident that while AI can strain your resources and contribute to environmental issues, it also holds the potential to be a force for positive change when harnessed responsibly and sustainably.
Mitigating Environmental Impact
Understanding the environmental challenges posed by developing and deploying AI systems is essential, but equally vital is exploring strategies to mitigate their impact. This section delves into various approaches for achieving sustainable AI.
Energy-Efficient Algorithms
One effective way to reduce AI’s environmental impact is through the development and adoption of energy-efficient algorithms. By optimizing the software that powers AI systems, you can decrease the computational demands, resulting in lower energy consumption during training and inference. Researchers and developers are actively working on creating algorithms that deliver the same or better performance with fewer computational resources.
Renewable Energy Usage
To make AI more environmentally friendly, organizations are increasingly powering their data centers and AI infrastructure with renewable energy sources like solar, wind, and hydropower. This shift toward clean energy helps reduce the carbon footprint associated with AI computations. Tech giants are leading the way by committing to using 100% renewable energy for their data centers and AI operations.
Hardware Efficiency Innovations
Innovations in hardware design and efficiency play a crucial role in mitigating the environmental impact of AI. This includes developing specialized AI chips that are more energy-efficient and improving the overall efficiency of AI hardware infrastructure. Energy-efficient hardware reduces the electricity needed for AI training and inference, contributing to a greener AI ecosystem.
Policy and Regulatory Efforts
Governments and regulatory bodies worldwide are recognizing the need to address the environmental impact of AI. This subsection discusses policy and regulatory measures aimed at promoting sustainable AI development and deployment.
Carbon Pricing and Taxation
Some regions are exploring carbon pricing mechanisms and taxation policies to hold organizations accountable for their carbon emissions, including those generated by AI operations. These financial incentives encourage companies to adopt cleaner AI technologies.
Energy Efficiency Standards
Regulatory bodies are developing energy efficiency standards specifically tailored to AI hardware and infrastructure. Compliance with these standards ensures that AI systems meet certain eco-friendly criteria, fostering responsible AI development.
Data Center Environmental Regulations
Environmental regulations targeting data centers and their energy sources are emerging. These regulations aim to limit the environmental impact of data centers and promote the use of renewable energy.
By implementing these strategies and supporting policy and regulatory efforts, you can move toward a more sustainable future for AI. These approaches not only mitigate the environmental impact of AI but also promote responsible innovation and environmentally conscious practices in the development and deployment of AI systems.

Photo: Kacper Pempel
Future Prospects
The future of AI holds promise for addressing the environmental impact associated with developing and deploying AI systems. This section explores emerging trends and initiatives in the realm of sustainable or “Green AI.”
Quantum Computing
Quantum computing is on the horizon as a game-changer in AI. Unlike classical computing, quantum computers use quantum bits (qubits) and have the potential to solve complex AI problems more efficiently. This could lead to significant reductions in energy consumption for AI training and inference, revolutionizing the environmental impact of AI systems. As quantum computing matures, its integration into AI could be a pivotal step toward greener AI solutions.
AI Ethics and Sustainability
The intersection of AI ethics and sustainability is becoming increasingly important. Ethical considerations in AI development include assessing the environmental impact and social responsibility of AI systems. Organizations are recognizing the need to integrate ethical principles into AI projects, ensuring that they align with sustainability goals. Initiatives focusing on AI ethics and sustainability aim to strike a balance between innovation and environmental responsibility, reinforcing the idea that AI should be a force for good.
As you look ahead, the integration of emerging technologies like quantum computing and a growing emphasis on AI ethics and sustainability offer hope for a more environmentally conscious AI landscape. These developments reflect a maturing understanding of the interconnectedness of technology, ethics, and your planet’s well-being. In the coming years, they will play a pivotal role in shaping the future of AI systems and their environmental impact.
Final Thoughts
It’s evident that while these systems have the potential to transform industries and improve lives, they also carry a significant environmental footprint. Your exploration of the environmental impact of developing and deploying AI systems has revealed both challenges and opportunities.
From the energy-intensive demands of AI training to the rapid turnover of hardware contributing to e-waste, environmental concerns are real and pressing. However, the good news is that the AI community is not oblivious to these issues. Strategies for sustainability, from energy-efficient algorithms to renewable energy usage, are actively being pursued. Policymakers are stepping in with regulations to encourage responsible AI practices, and emerging technologies like quantum computing offer exciting prospects for greener AI solutions.
In this balancing act between innovation and environmental responsibility, the key takeaway is clear: AI must be developed, deployed, and regulated with a keen eye on its environmental impact. As you move forward, the path to a more sustainable AI ecosystem is within reach, where AI systems are not just smart but also environmentally conscious, ushering in a brighter and greener future for all.
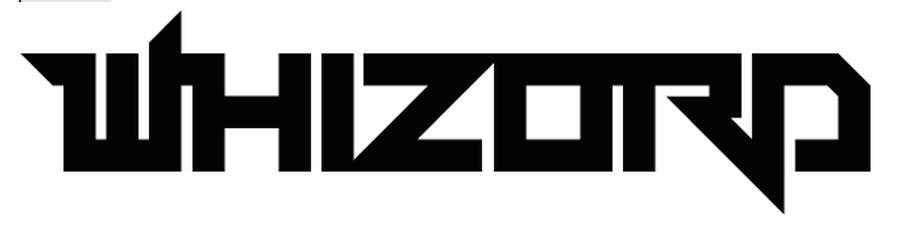