- Player segmentation
- Predictive modeling
- Procedural content generation
- Pathfinding

Photo: Florence Lo

Photo: Alena Darmel
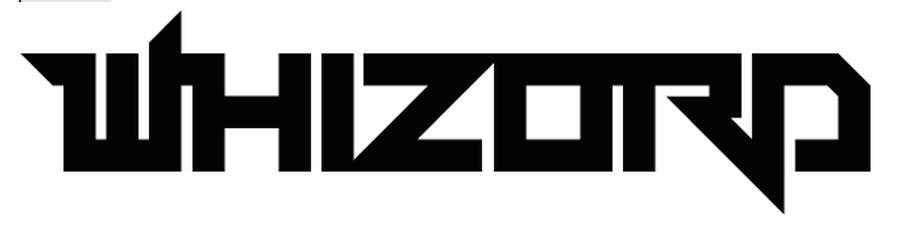
Hi, what are you looking for?
Photo: Florence Lo
Photo: Alena Darmel
Elon Musk’s influence on U.S. government technology sparks controversy, as Musk-inspired strategies disrupt the federal tech sector. Unorthodox practices, weakened oversight, and dismantled DEIA...
Elevate your home with Design Within Reach’s winter sales event, featuring up to 60% off clearance items, flash sales, and an exclusive "EXTRA20" promo...
Samsung's February 2025 deals offer major savings on cutting-edge tech, including 30% discounts for community heroes, bundle offers like $4,500 off a 98-inch QLED...
Amazon Music Unlimited will raise subscription prices in early 2025, citing enhanced features like audiobook borrowing and a personalized year-in-review tool. Prime members face...