Preface
Predictive analytics: A Definition and Significance for Cybersecurity
In cybersecurity, predictive analytics indicates a paradigm exchange from reactive to proactive safety tactics. Predictive analytics makes use of statistical models and complicated algorithms to hit upon viable risks earlier than they occur, giving firms the opportunity to patch vulnerabilities ahead of time. This proactive strategy is important in a time whilst cyberthreats are getting greater complex and persistent.
Historical Context: Cybersecurity Measures’ Evolution
The field of cybersecurity has modified drastically inside the last several a long time. It commenced with a focus on firewalls and simple virus protection, however it has grown to encompass state-of-the-art network defenses, intrusion detection structures, and endpoint safety. The improvement is a reflection of the growing complexity of cyberthreats, which calls for greater sophisticated and flexible defenses.
Present Cybersecurity Trends and Challenges
The cutting-edge nation of cybersecurity is characterized by means of quickening technological development and a developing assault surface because of the considerable use of cloud services and related gadgets. Many firms battle to locate and reply to threats in a timely way in spite of large investments, often because of the quantity of facts and sophisticated attack techniques used by cybercriminals.
Gaining Knowledge of Predictive Analytics
Predictive analytics: An Overview and Components
Utilizing statistical methods, system gaining knowledge of algorithms, and historic information, predictive analytics makes predictions about what will appear in the destiny. The accumulating and preparation of records, the creation and validation of the model, and the implementation for actual-time prediction are critical factors. These models use ancient records to identify tendencies and foresee viable risks.
How Traditional Data Analysis and Predictive Analytics Differ
Conventional information evaluation addresses what came about and why by way of emphasizing diagnostic and descriptive findings. Predictive analytics, on the other hand, seeks to decide what is most in all likelihood to occur next. This proactive method is in particular beneficial in cybersecurity, in which primary harm may be avoided by foreseeing and keeping off dangers before they materialize.
Important Technology for Predictive Analytics
Predictive analytics is primarily based on a number of technologies, such as records mining, large information, synthetic intelligence, and system studying. Large volumes of statistics can be processed and analyzed in real time using those technologies, which additionally make it feasible to find hidden connections and styles that might factor into capability dangers.
Predictive Analytics’s Place in Cybersecurity
Prompt Threat Identification and Mitigation
Early hazard detection is substantially improved by way of predictive analytics, which continuously video display units network activity and user behavior to spot abnormalities. It can perceive potentially risky styles early on and alert cybersecurity professionals to do so before any real damage is completed via spotting anomalous styles that diverge from the norm.
Behavior Analysis and Identification of Anomalies
A vital aspect of cybersecurity predictive analytics is conduct analysis. Predictive fashions identify anomalies that would imply malicious interest via placing baselines for traditional person and gadget conduct. This approach assists in recognizing complicated attacks and insider threats that conventional approaches should forget about.
Cutting Down on False Positives and Increasing Reaction Times
The excessive percentage of false positives, which could overload the safety workforce and cause warning fatigue, is one of the important difficulties in cybersecurity. Predictive analytics reduces false positives with the aid of optimizing detection algorithms, which increases accuracy. This development makes it viable to reply to real threats quicker and more effectively.
Important Tools and Technologies
Botanical intelligence and device studying
Predictive analytics is based on synthetic intelligence (AI) and system gaining knowledge of (ML). These technologies deliver systems the potential to learn from records and step by step enhance their capacity to come across threats. Artificial intelligence (AI)-powered cybersecurity answers provide strong and bendy defenses by means of routinely adapting to emerging threats.
Data mining and massive data
Large volumes of records from numerous assets can be processed and gathered more easily thanks to huge data technology. After that, full-size patterns and correlations are extracted using facts mining tools. In cybersecurity, this includes combining data from user interest logs, network logs, and danger intelligence feeds to create thorough threat models.
Systems for Advanced Threat Protection
Predictive analytics is incorporated into superior danger safety (ATP) structures to offer a comprehensive protective method. These structures provide real-time threat identification, evaluation, and reaction by means of combining large records, gadget mastering, and synthetic intelligence. They have the capability to apprehend and counter state-of-the-art threats like superior continual threats (APTs) and zero-day exploits.
Case Studies: Effective Applications
Predictive analytics has been efficaciously included into cybersecurity strategies by way of numerous corporations. For instance, a large financial organization reduced financial losses significantly via using predictive fashions to identify and forestall fraud. An similar example involves a healthcare provider utilizing predictive analytics to safeguard affected person facts and guarantee adherence to policies.
Predictive analytics’s advantages for cybersecurity
Preventive Threat Control
A proactive technique to cybersecurity is made feasible by means of predictive analytics, which moves the emphasis from responding to incidents to keeping off them. Organizations can reduce the effect of attacks and safeguard their assets by means of proactively detecting possible risks.
Improved Precision and Effectiveness
Threat detection systems operate greater correctly and efficiently when predictive analytics is used. These systems get better at recognizing real threats through constantly enhancing detection fashions with fresh records. This lowers the variety of fake positives and frees up the protection body of workers to pay attention to real threats.
Resource optimization and fee discount
By warding off steeply-priced breaches and making the excellent use of cybersecurity assets, the software of predictive analytics can result in massive cost financial savings. By getting rid of the need for guide involvement, automatic threat detection and response frees up safety staff to concentrate on strategic initiatives and tricky risk evaluation.
Difficulties and Restrictions
Concerns approximately Data Privacy and Ethics
Large datasets are utilized in predictive analytics, which creates ethical and records privacy problems. It is imperative for organizations to adhere to data protection standards and manage confidential data with due diligence. Furthermore, great thought needs to be given to the ethical ramifications of automated decision-making in cybersecurity.
Connecting with Current Systems
It can be difficult to integrate predictive analytics with the current cybersecurity framework. Advanced analytics tools might not be supported by legacy systems, necessitating major upgrades or replacements. Proper integration and interoperability are essential for predictive analytics to be deployed effectively.
Training Requirements and Skill Gaps
Predictive analytics specialists in the cybersecurity field are in high demand. Investing in training and development programs is vital to close the skill gap and provide security teams with the requisite knowledge and abilities. Establishing a culture of perpetual learning is crucial for organizations to stay up to date with technological changes.
Prospective Patterns and Forecasts
Developments in AI and Machine Learning
Predictive analytics in cybersecurity will continue to advance due to developments in AI and machine learning. Deep learning and reinforcement learning are two examples of emerging technologies that hold promise for improving threat detection capabilities and enabling more complex security tactics.
IoT and cloud security integration
As the threat surface grows, the integration of predictive analytics with IoT and cloud security will become more and more crucial. When it comes to tackling the particular difficulties presented by cloud-based services and linked devices, predictive models will be essential.
The Changing Environment of Threats
The threat landscape is ever-changing, and cybercriminals are using ever-more-advanced tactics. To keep ahead of attackers and safeguard important assets, predictive analytics will need to adjust to these developments and make use of the most recent technology developments.
Predictive Analytics in Business Intelligence
Customisation and control
By its nature, predictive analytics gives users a platform to personalize and customize products and services. Businesses will have much greater control with the ability to anticipate needs and the capability to deliver tailored experiences.
Long gone are the days of marketing to broad segments of similar customers, businesses are enabled to have sophisticated campaign execution that creates a one-to-one dialogue with consumers in real-time on any channel.
Greater diversification
Right now, much of the predictive analytics activity has been focused on how customer behavior today will impact the way in which they spend money in the future. However, many additional applications are being developed and in the coming years we will see huge diversification in this space.
This will include application of predictive analytics to help companies beyond consumer revenue generation to all business areas, such as HR for tasks like recruitment to find the right candidates or monitoring patterns in employee health and wellbeing for productivity. We’ll also see this extend into smaller and more niche industries.
Integration with IoT
Sensors are flooding the marketplace, from mobile phones, automated homes to traditional manufacturing processes. Devices and sensors are able to collect vast amounts of data related to consumer interactions, products on a supply chain, environmental conditions and so on.
How this information is interpreted to meet customer expectations, carry out preventive maintenance, optimize processes etc. is a key battleground for businesses looking for success in tomorrow’s marketplace.
Supply and demand
As any economist will tell you, affordability and availability of a product or service is directly proportional to the number of people embracing it.
Right now, predictive analytics is relatively accessible, but as the business benefits are better understood, the pace of adoption for predictive analytics will grow from isolated pockets to enterprise wide organizational deployment. This will mean that relying on predictive analytics will become commonplace for business leaders of all sizes.
Data visualization
Raw data is hard to monitor and interpret, even for experienced data analysts. That’s why data visualization is such a hot rising trend; predictive analytics will soon take giant steps forward into projecting data in a more visual format, helping users gain intuitive takeaways and more easily communicate their conclusions.
The big and small picture
Predictive analytics tends to focus on the macro, big picture insights, trends and high-level takeaways – important for business consumption, but as the ability to perform predictive analytics at scale evolves, businesses will be able to dig much deeper when it comes to predicting the micro movements and behaviors of clients and customers.
Looking ahead
The entire business analytics framework, from data ingestion through to delivery, is going to evolve to become more equipped to generate real-time insights at the point of work, provide automation for mundane daily tasks, and suggest actions for business users.
This is all good. Yet, in order to remain competitive, businesses must be looking toward building predictive analytic capabilities as this is the future for data driven business, and those that aren’t investing will lag behind the competition
Common Questions on Cybersecurity Predictive Analytics
1. What is Cybersecurity Predictive Analytics?
Cybersecurity predictive analytics uses stats, ML, and past data to anticipate and prevent cyber threats before they occur. By locating and fixing vulnerabilities early on, this proactive strategy aids enterprises in improving their security posture.
2. How does cybersecurity get better using predictive analytics?
By facilitating early threat detection, decreasing false positives, and speeding up response times, predictive analytics enhanced cybersecurity. Predictive models can spot possible dangers and offer useful information for preventative action by examining trends and anomalies in data.
3. In cybersecurity, what are the primary obstacles to integrating predictive analytics?
Predictive analytics in cybersecurity faces hurdles like data protection, ethics, system integration, and skill gaps. Companies need to overcome these obstacles in order to use predictive analytics for improved cybersecurity.
Key Takeaway
Synopsis of Key Ideas
- With its proactive approach to threat identification and prevention, predictive analytics is the next frontier in cybersecurity.
- Organizations boost security and stay ahead of risks by leveraging AI, ML, and big data technologies.
- Predictive analytics has significant advantages in terms of accuracy, efficiency, and cost reductions despite its difficulties.
The Value of Keeping Up with Cybersecurity Developments
- Being ahead of the curve in cybersecurity is crucial in an era of growing cyberthreats. Organizations secure assets and trust in digital realms using predictive analytics to anticipate and mitigate potential risks.
- Building strong and resilient cybersecurity defenses requires investing in predictive analytics and resolving related issues.
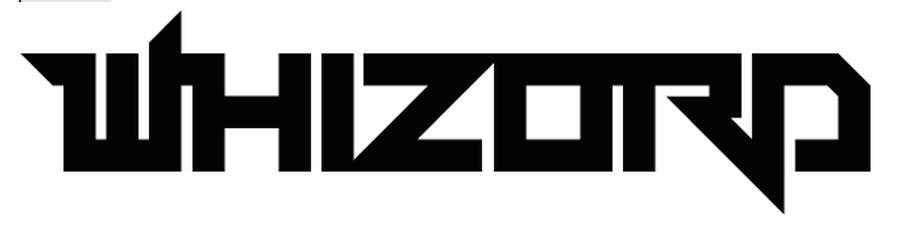